CrowdDNA targeted breakthroughs
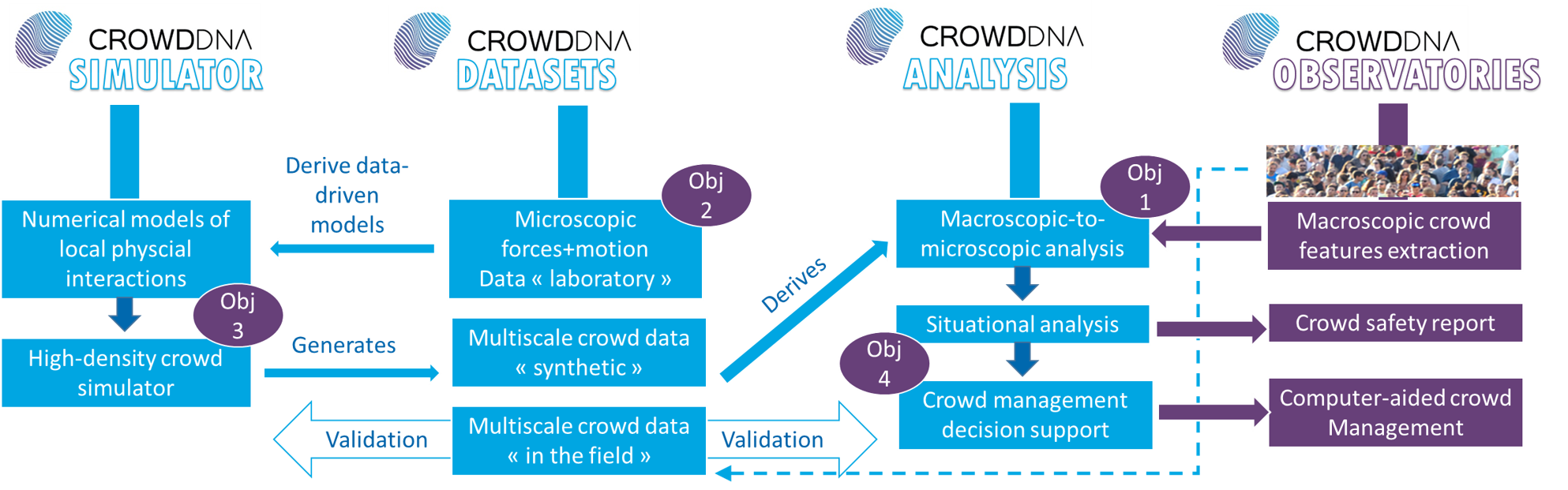
As a proof of concept, CrowdDNA intends to implement and to deliver the architecture illustrated in this figure:
CrowdDNA Simulator will advance the field of crowd simulation by introducing a yet unexplored scale of modelling of local interactions, down to limb-to-limb interactions, which is required to predict the effect of contacts and pushing between people, the emergent crowd movements, the levels of pressure on human bodies, or the risks of fatalities, among others.
CrowdDNA Analysis will advance the field of crowd motion analysis, by introducing new paradigms that can lead to predictive analysis in real-time, detect dangerous crowd configurations before any uncontrolled movement appear, and give a chance to take crowd management decisions and actions before dangerous events occur.
CrowdDNA Datasets is essential to the achievement of CrowdDNA targeted breakthroughs, and will represent a major contribution to the field of Crowd Science beyond CrowdDNA, as they will enable the community at large to explore the challenging question of scale coupling in crowd behaviours.
CrowdDNA Observatories consists in establishing long-term collaboration with mass event organizers, crowd management companies or public buildings operator to demonstrate the prototypes resulting from CrowdDNA, to face the reality of live conditions for crowd management, as well as to evaluate and to validate our approaches.
Fields
Physics
Cognitive Science
Computer Science
Machine Learning
Crowd Management
Crowd Simulation
CrowdDNA gathers researchers partners from the fields of Physics, Cognitive Science, Computer Science and Machine Learning together with SMEs in the field of Crowd Simulation and Crowd Management, in order to boost crowd science and develop the revolutionary techniques needed to derive the technological solutions sought.
Objectives
The overall objective is to develop
This raises the 4 following specific objectives:
The approach taken by CrowdDNA is fundamentally based on the idea that a given crowd configuration will, in a bottom-up emergent process, provoke specific physical interactions between people and lead to specific crowd movements.
Objective O1 is to make us capable of inverting these bottom-up relations to infer crowd configuration and level of intensity of physical interactions from some observable global crowd movement features.
This is a general problem in the field of crowd science: understanding how the microscopic scales are coupled with macroscopic scales of crowd behaviours. Nevertheless, CrowdDNA aims at exploring new relations based on a Machine Learning approach and addresses radically new challenges.
The previous objective requires to dispose of crowd datasets that simultaneously describe local exchanges of forces together with larger scale motion data (individual, group or crowd motions). Such a dataset does not exist yet especially because of the lack of technologies to achieve it: the missing brick is force capture.
CrowdDNA objective O2 is to decipher the mechanisms of crowd behaviours at those different scales. We need to study the dynamics of a system formed by few individuals exchanging forces (standing close, moving, pushing, etc.).
What are the body surfaces in contact? How are forces exchanged between people? How do individuals react and generate internal forces by themselves? How do the location of pushes on the body, the predictability of forces, or the underground structure affect body balance and thus the risk of falling? Answering those questions raise a big challenge in terms of experimental methodology that CrowdDNA will raise based on cutting-edge approaches from Biomechanics and musculo- skeletal modelling.
CrowdDNA objective O2 provides essential observations of dense crowd behaviours. However, to achieve objective O1, we need to study these interactions in all imaginable scenarios, which can never be fully covered by a real-world dataset. To palliate this, we intend to derive numerical models of local physical interactions from the O2 dataset.
The objective of such model to establish the relations between the internal states of simulation agents (motion, balance, etc.) with the external constraints applied to them (pushes, contacts, obstacles, etc.). Models of local interactions are then immersed at the core of a crowd simulator to predict how large crowds behave in specific scenarios.
The major associated challenge is scalability. It would be impossible to numerically detail and simulate the geometries and the physical interactions between numerous bodies. Therefore, CrowdDNA intend to explore new machine learning techniques to derive models from force + motion data (O2).
Based on O1-O3, O4 aims to predict imminent dangers and compute practical guidance to alter crowd configurations to avoid disastrous situations. The practical guidance will be specific and implementable to both authorities and crowd members, e.g. advising the authority to reduce the density of certain areas, and/or informing individuals to move in certain directions (similar to a navigation map) to disperse crowds in a coordinated manner.
CrowdDNA will achieve O4 by first computing the danger/discomfort of the current crowd configuration and its likely variations of evolvements in the near future (O3). This considers both the high-level features and the inferred low-level interactions (O1). If it identifies any potential danger, it will compute a safe configuration as the target configuration, together with a crowd-morphing plan.
O4 is one main reason why CrowdDNA is deeply more radical than existing methods. The resulting crowd model can provide solutions that have immediate and direct real-world impact on real crowds. CrowdDNA will be superior to human-experience-based methods and current technologies.
The approach taken by CrowdDNA is fundamentally based on the idea that a given crowd configuration will, in a bottom-up emergent process, provoke specific physical interactions between people and lead to specific crowd movements. Objective O1 is to make us capable of inverting these bottom-up relations to infer crowd configuration and level of intensity of physical interactions from some observable global crowd movement features. This is a general problem in the field of crowd science: understanding how the microscopic scales are coupled with macroscopic scales of crowd behaviours. Nevertheless, CrowdDNA aims at exploring totally new relations based on a Machine Learning approach and addresses radically new challenges.
The previous objective requires to dispose of crowd datasets that simultaneously describe local exchanges of forces together with larger scale motion data (individual, group or crowd motions). Such dataset does not exist yet especially because of the lack of technologies to achieve it: the missing brick is force capture. CrowdDNA objective O2 is to decipher the mechanisms of crowd behaviours at those different scales. We need to study the dynamics of a system formed by few individuals exchanging forces (standing close, moving, pushing, etc.). What are the body surfaces in contact? How are forces exchanged between people? How do individuals react and generate internal forces by themselves? How do the location of pushes on the body, the predictability of forces, or the underground structure affect body balance and thus the risk of falling? Answering those questions raise a big challenge in terms of experimental methodology that CrowdDNA will raise based on cutting-edge approaches from Biomechanics and musculo- skeletal modelling.
CrowdDNA objective O2 provides essential observations of dense crowd behaviours, we however need to study them in any imaginable scenarios that no dataset could completely cover to achieve O1. To palliate this, we intend to derive numerical models of local physical interactions from the O2 dataset. The objective of such model to establish the relations between the internal states of simulation agents (motion, balance, etc.) with the external constraints applied to them (pushes, contacts, obstacles, etc.). Models of local interactions are then immersed at the core of a crowd simulator to predict how large crowds behave in specific scenarios. The major associated challenge is scalability. It would be impossible to numerically detail and simulate the geometries and the physical interactions between numerous bodies. Therefore, CrowdDNA intend to explore new machine learning techniques to derive models from force + motion data (O2).
Based on O1-O3, O4 aims to predict imminent dangers and compute practical guidance to alter crowd configurations to avoid disastrous situations. The practical guidance will be specific and implementable to both authorities and crowd members, e.g. advising the authority to reduce the density of certain areas, and/or informing individuals to move in certain directions (similar to a navigation map) to disperse crowds in a coordinated manner. CrowdDNA will achieve O4 by first computing the danger/discomfort of the current crowd configuration and its likely variations of evolvements in the near future (O3). This considers both the high-level features and the inferred low-level interactions (O1). If it identifies any potential danger, it will compute a safe configuration as the target configuration, together with a crowd-morphing plan. O4 is one main reason why CrowdDNA is deeply more radical than existing methods. For the first time to our knowledge, a crowd model can provide solutions that have immediate and direct real-world impact on real crowds. CrowdDNA will be superior to human-experience-based methods and current technologies.