D3.1: ML Generator to analyse crowd movements in videos
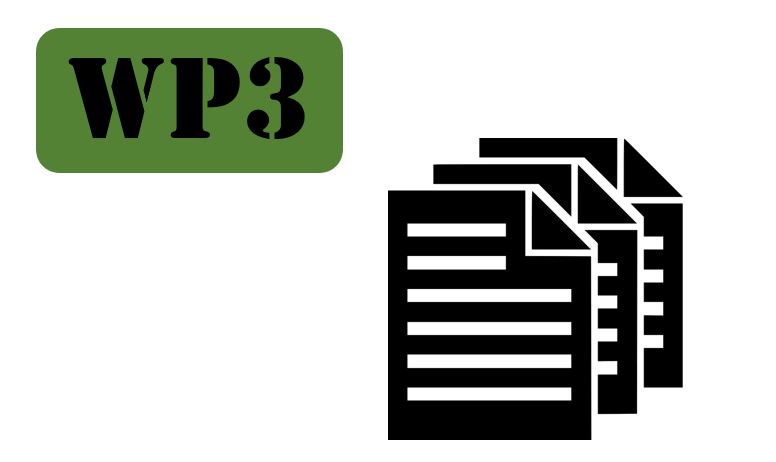
This deliverable describes the efforts done during periods 1, 2 and half-way through 3 in the Work Package 3 (WP3) of the project towards, first, generating synthetic data and, second, training a detector. As a reminder, the overall objective of CrowdDNA WP3 is to deliver algorithms to detect micro-scale information of human interactions from macro scale observations such as crowd videos.
To this end, the consortium has worked on several key ingredients that leverage the crowd simulator described in Deliverable 2.1 to create synthetic data and annotations regarding human contact in dense crowds. In particular, we have extended our simulator to resolve the collisions that emerge when articulated 3D crowds are synthesized from 2D trajectories. Detecting and resolving collisions is a fundamental part of WP3, because it enables the computation of micro-scale inter-human interactions (e.g., pushes, contacts, etc.). Additionally, we have showed that collision-aware 3D crowds can also be obtained from 2D trajectories captured in the real world (i.e., not simulated) and, more specifically, we have used data captured within the project in WP1 at FZJ facilities to train our models.
Data generated is used to train a machine learning model capable of inferring micro scale properties of a crowd observed from a surveillance camera. Importantly, we have tested our approach in real-world video footage, demonstrating that our solution generalizes well to unseen conditions.
CrowdDNA © 2021. All rights reserved. | Designed by ArtificialIdeas
This project has received funding from the European Union’s Horizon 2020 research and innovation program under grant agreement No 899739.
Cookie | Duration | Description |
---|---|---|
cookielawinfo-checkbox-analytics | 11 months | This cookie is set by GDPR Cookie Consent plugin. The cookie is used to store the user consent for the cookies in the category "Analytics". |
cookielawinfo-checkbox-functional | 11 months | The cookie is set by GDPR cookie consent to record the user consent for the cookies in the category "Functional". |
cookielawinfo-checkbox-necessary | 11 months | This cookie is set by GDPR Cookie Consent plugin. The cookies is used to store the user consent for the cookies in the category "Necessary". |
cookielawinfo-checkbox-others | 11 months | This cookie is set by GDPR Cookie Consent plugin. The cookie is used to store the user consent for the cookies in the category "Other. |
cookielawinfo-checkbox-performance | 11 months | This cookie is set by GDPR Cookie Consent plugin. The cookie is used to store the user consent for the cookies in the category "Performance". |
viewed_cookie_policy | 11 months | The cookie is set by the GDPR Cookie Consent plugin and is used to store whether or not user has consented to the use of cookies. It does not store any personal data. |